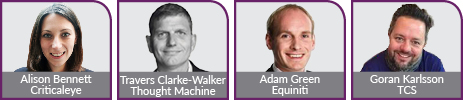
Don’t be fooled into thinking artificial intelligence is a futurist fantasy. A study by Tata Consultancy Services (TCS) found that 91 percent of European companies are already using AI and 57 percent see it as essential for global competitiveness.
By 2020, the majority of those companies expect to be using AI as a means to transform their company, yet according to Goran Karlsson, Digital Champion of AI and Cognitive Solutions at TCS, more should be done.
"In my profession there is a lot of optimism about how AI will be able to transform businesses. That said, the majority of companies aren’t making bold investments in it. This will create a competitive imbalance versus the companies that are already not only investing, but really understanding the full business benefits of AI," he says.
“We should embrace AI, yet also educate ourselves on its implications,” says Alison Bennett, Relationship Manager at Criticaleye, who works with business leaders employing this technology.
“Corporates must examine how their businesses can prosper from this technology, while protecting against risks such as hacking. The general populous, on the other hand, should consider things like their own data privacy.”
Here, we examine four of AI’s major implications.
Cool versus Creepy
Imagine you’re about to buy a coffee, it’s your third that day. Your phone, noting your location, sends a message suggesting you put the £2.70 you were about to spend into your pension scheme instead. This scenario is just one way in which Travers Clarke-Walker, SVP of Sales and Marketing at Thought Machine, imagines AI can help us to make better decisions.
“We hear a lot of negatives about AI, for example that algorithms have decided you’re not viable for an insurance product or loan, but it can also be used to improve your financial literacy. In the not-too-distant future, I could analyse how much I’m spending on food and how healthy it is, then share that information with my insurance provider or bank in return for a better deal.”
Some people are understandably concerned about how such tools might invade personal privacy. “There is a key requirement for institutions to learn the balance between what’s creepy versus supportive, and I think that’s down to the manner in which it’s delivered,” says Travers.
“If someone approaches you knowing a load of information about you without it being immediately obvious or observable how they know it, that’s creepy. If, on the other hand, they explain that they’ve taken the time to get to know you in order to express a view or offer something that’s pertinent to your personality, that’s probably a good thing.”
Escaping the Echo Chamber
Many of today’s news, financial reports and press releases are written by AI-fuelled systems, which even decide what content reaches us. For example, Facebook’s algorithms filter content, pushing out adverts and information that are demographically relevant to the reader. While this selection process improves personalisation, it also creates what’s being termed as an ‘echo chamber’ – an enclosed space in which the same views and information continuously bounce off each other.
“Facebook has an incredible power to limit your horizons. What you see on it is a very narrow band, not just of life but your experience of it,” says Adam Green, Chief Risk Officer at Equiniti, noting that it could have long term social implications.
“You could end up in moral quagmires as this provides an opportunity for propaganda. It’s one thing to bring a message to somebody, it’s another to then use the information you have about them to systematically bend their outlook. The question is how far we should allow that.”
Travers adds: “Narrowing that person’s field of vision is actually not very observable to the individual; you don’t know it's happening to you. I do think that organisations have some ethical responsibility to expose people to the opportunity to learn more than they already know.”
The Imperfect Algorithm
It’s surprising how much we already rely on AI. Take BlackRock’s risk management super computer, Aladdin, which is employed by some 60 financial firms in managing seven percent of the world’s total wealth. While incredibly effective, it has raised concerns about the risk of unreservedly trusting its output.
“After the financial crisis, I spoke with a range of boards about how to rethink financial risk models. Some financial services projections and decisions were based on models that were broadly taken at face value and there was a notable absence of challenge. It was only after the crisis that there was a widespread realisation the models had inherent limitations. For example, some securitised mortgage models did not include parameters to reflect that house prices could decline,” says Adam.
As AI becomes more adept, it also becomes more complex making it harder for humans to understand, let alone challenge. Computers are now able to iteratively create their own algorithms – continuously perfecting for months on end, meaning the finished model is unintelligible to humans.
“To start to understand a complex model you should systemically look at the inputs and outputs, especially around unexpected events. Many complex or machine learnt modes are very much a black box – yet the knowledge and discipline to recognise that and treat them cautiously is still not consistently available,” says Adam. “We need to retain control and a reasoned understanding of model outputs.”
One solution already in development is LIME, short for Local Interpretable Model-agnostic Explanations. It analyses the calculations made by existing AI models, translating them into information that’s understandable to humans. Those people then challenge any discrepancies or assumptions in the original model and edit its decision-making process.
Challenging Bias
“Naïve AI, such as an algorithm or system that simply digests and replays the world around us, is always limited by what we feed it,” notes Travers.
Our biased world is then reflected in biased statistical assumptions. One example comes from the independent US newsroom, ProPublica, which found racial bias in an algorithm employed by the US justice system to calculate the likelihood of an individual re-offending. That biased statistic has been used by Judges to set sentences.
“If you start structuring the machine to be anti-bias, that’s a bias in itself, and so the question is, how ethical is that?” asks Travers.
Bias is therefore another reason for regulatory oversight, Travers argues. “I think it’s important that there is some degree of assessment, insuring that everyone has free, liberalised access to products and services plus the ability to challenge the outcome of AI-generated decisions. When you remove the opportunity to react against the answer, you’ve fundamentally breached the principles of free access to products, services and markets.”
By Mary-Anne Baldwin, Corporate Editor, Criticaleye
These thoughts were shared during Criticaleye’s recent events on The Implications of AI and Why Artificial Intelligence Will Transform your Business
For further insights on AI, check out How Artificial Intelligence is Revolutionising HR & Tomorrow's Workforce. Don't miss upcoming Conference Call The Board's Role in Curbing Cyber Crime & Criticaleye Asia Member Meeting Driving Business through Digital.
For further insights on AI, check out How Artificial Intelligence is Revolutionising HR & Tomorrow's Workforce. Don't miss upcoming Conference Call The Board's Role in Curbing Cyber Crime & Criticaleye Asia Member Meeting Driving Business through Digital.